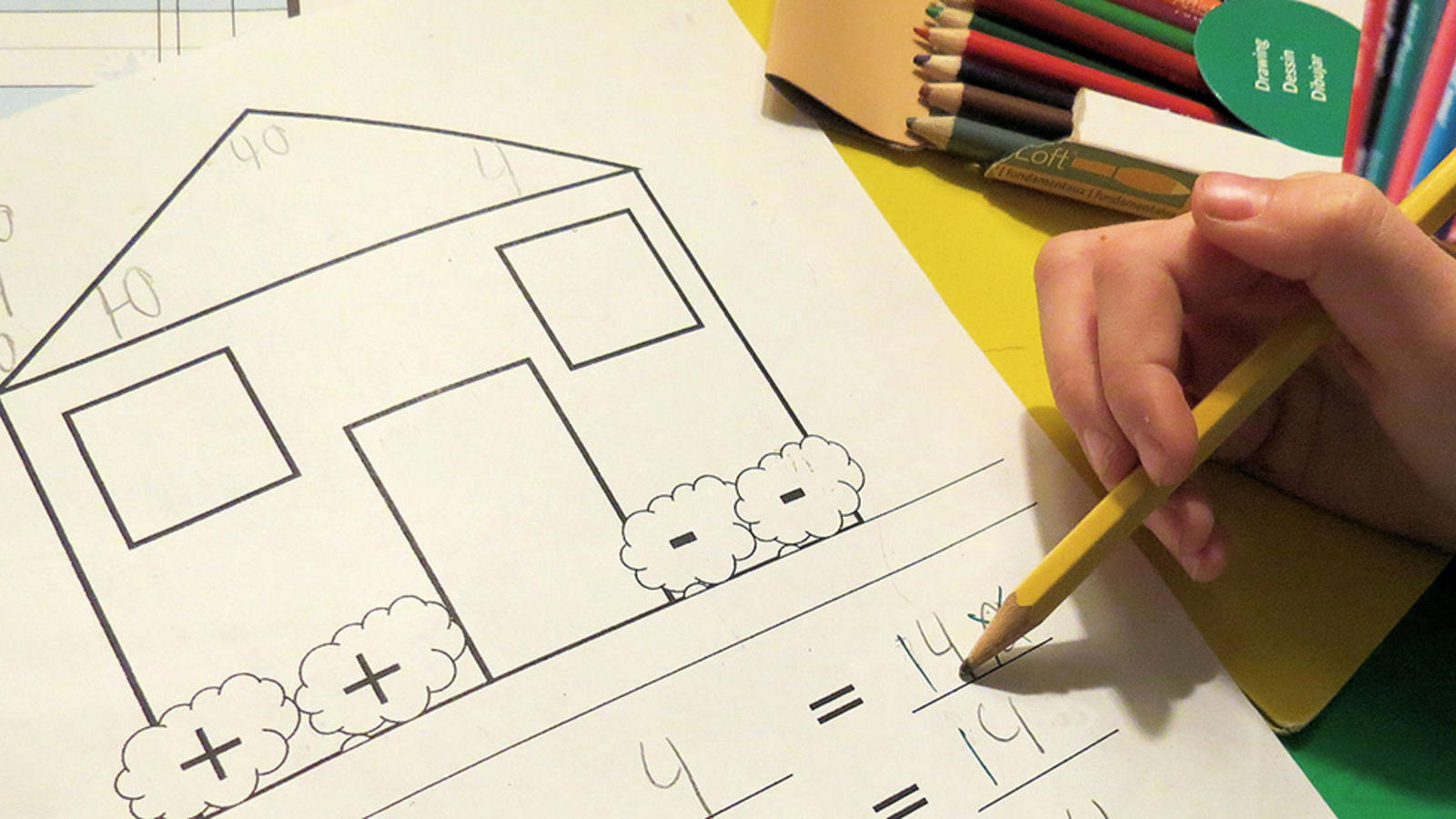
Miscount
Many Tests, Big Conclusions: What Could Possibly Go Wrong?
Based on research by David Lane
Many Tests, Big Conclusions: What Could Possibly Go Wrong?
- Researchers need to avoid intense focus on subgroups when the big picture doesn’t support their conclusion.
- Readers of numbers based research and reporting should always be aware of the sample sizes studied.
- Journalists who report on statistics based research should consider taking statistics courses.
Most people know at least a little about the signs of the zodiac, and the supposed characteristics of those born under them. Aries are brave; Cancers tenacious; Scorpios resourceful. But did you know that Sagittarians are prone to funny-bone fractures? Or that Leos tend to be plagued by stomachaches?
It all sounds like nonsense, you say — especially the part about diseases and injuries being linked to astrological signs. But according to David Lane, an associate professor of statistics and management at Rice, those indeed were the findings of a 2006 study that classified patients according to their signs, and then went looking for any differences in incidence of particular ailments.
Don’t get Lane wrong, though. He in no way defends the notion that diseases and star signs are linked. Neither did the authors of the original study, which was designed as a lesson in the perils of shoddy science. Instead, in an article critiquing the careless use of statistics, Lane warns that research that makes too many statistical comparisons boosts the chances of false conclusions.
In other words, if scholars conducting a study focus on too many subgroups — Geminis and Capricorns, say — rather than on the big picture, they're likely to get inaccurate, even nonsensical, findings.
These studies also pose a special hazard for science reporters. Lacking background in statistics and numeracy, such journalists can easily misunderstand studies that are statistically complex.
Lane uses the New York Times blog of Steve Lohr to illustrate his point. In 2010, the National Bureau of Economic Research published a report on the effectiveness of online education. Lohr quoted the report's authors, who wrote, “A rush to online education may come at more of a cost than educators may expect,” and generally conveyed skepticism about the “rush” to online college learning, especially regarding “certain groups” that “did notably worse online.”
Hispanics who studied online, these authors contended, scored a full letter grade lower than Hispanics who attended a live lecture. Males and low achievers each lost half a letter grade.
To the casual reader, this looked like bad news about online education overall. Readers who weren’t statistically savvy also might have concluded that Latino online learners fare especially badly, and that online classes don’t work as well as in-person teaching for anyone. But none of that was actually supported by the report’s findings.
According to Lane, the blog failed to convey a rather important fact: there is “no credible evidence" for the notion that live lectures have any better outcomes than online ones.
Even more misleadingly, the Times blog didn’t reveal that the sample size for the Hispanics viewing online lectures was only eight people. While the overall group tested was an adequate 312 students, Lohr failed to note the small number of Hispanics who were viewing online lectures.
In other words, the New York Times reported on the performance of Hispanic students in online classes based on a study that included only eight Hispanics in the critical group. “A proper comparison," Lane writes, "would take into consideration the large margin of error that necessarily accompanies the very small sample size used.”
Lane’s article sends up a warning about research findings based on subgroups to the exclusion of the big picture. It’s the scientific equivalent of believing that the cosmos revolves around the Big Dipper.
There’s good reason, in other words, to doubt that Sagittarians have fragile bones. Sound science requires a grasp of statistical complexity – and acknowledgment that the universe holds more than just the stars we can see.
David M. Lane is an associate professor in the departments of psychology, statistics and management at Rice University.
To learn more, please see: Lane, D.M. (2013). The problem of too many statistical tests: Subgroup analyses in a study comparing the effectiveness of online and live lectures. Numeracy, 6(1), Article 7.
Never Miss A Story